In Part 1 of a two-part series on how federal agencies can adopt improved data management programs, Joah Iannotta Senior Data Governance Expert at ABSG Consulting Inc., looks at the importance of combining policy with a well-planned compliance and evaluation program and the risk to an agency of not taking this approach.
-----------------------------------------------------------
Failing to ensure compliance with new requirements can magnify gaps and weaknesses for auditors, akin to writing the organization’s next audit findings and delivering them to its Inspector General with a neat bow on top.
RELATED
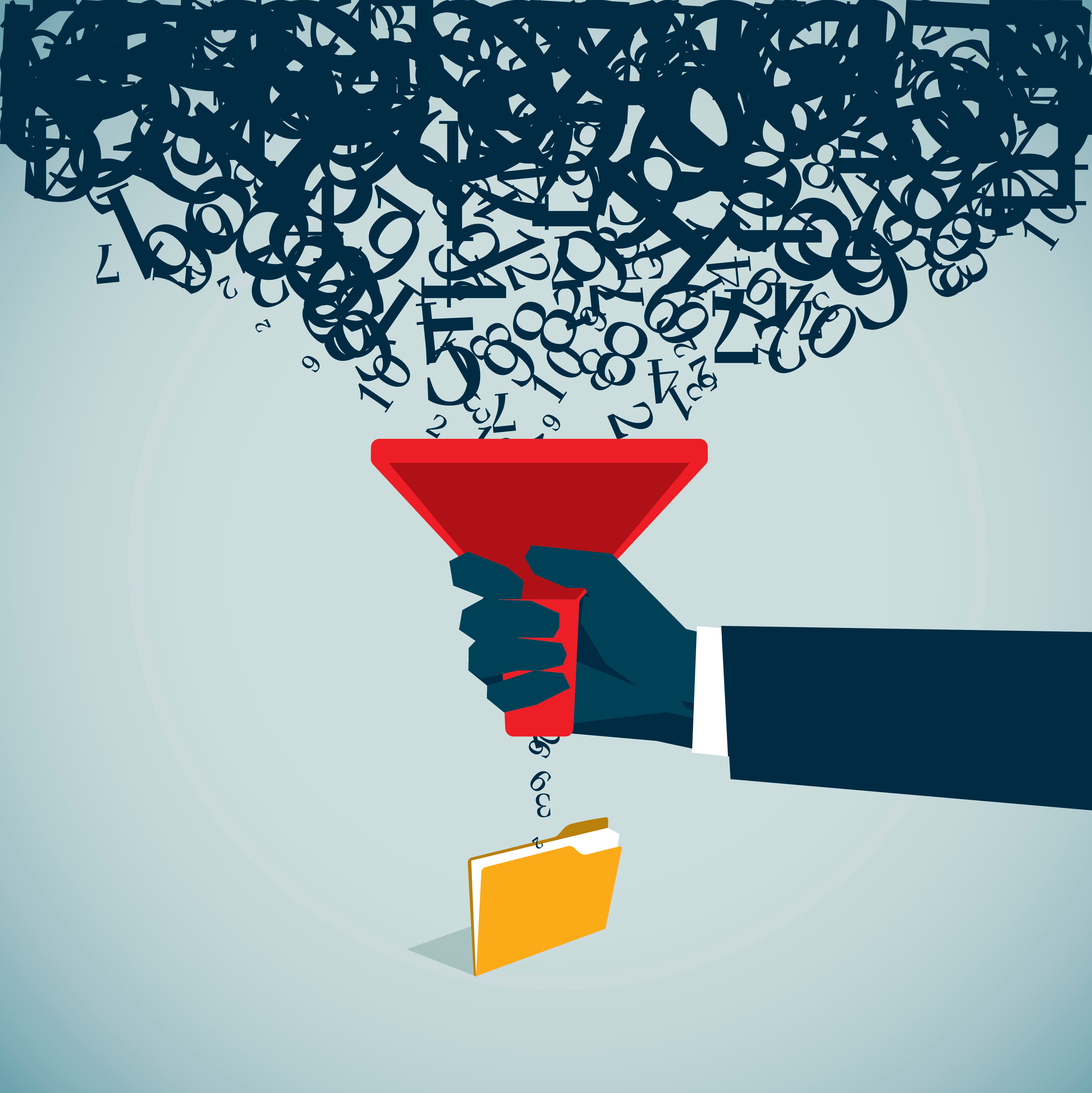
Agencies often view the creation of policy, directives, guidance, and instructions as a self-contained task. Policy shops are established, and their measure of success often is the publication of a number of policy documents. Once a policy is published, the lines of business are left to interpret the policy and develop implementation solutions from the ground up. When looking at the federal government, policy shops can tend to take a hands-off approach to implementation, on the basis that it is best to provide the program with maximum flexibility to implement policy in a way that best suits their organization.
This approach very much emulates the relationship between the federal government and states, where the federal government sets a policy and program directive but allows the states wide latitude for implementation. This approach allows states to innovate and test approaches that suit their demography, culture, and particular needs, which can result in successful pilot programs being adopted and adapted by other states.
However, this process can take time and result in failed experiments. While this type of incubation can be beneficial for creating large scale social and economic programs, it’s not necessarily the most efficient way to implement enterprise data governance policy.
Most organizations are driven to revamp or create a new enterprise data governance policy because there has been an abundance of innovation, new solutions, and problem-solving at the “State” level within the organization, with the agency beginning to feel the pain of the proliferation of data-oriented solutions.
Ungoverned data
For example, one source of pain is that, at a certain point, the proliferation of ungoverned data and data solutions can actually hinder an agency’s maturation towards becoming data-driven.
Consider two different grant programs designing solutions to capture applicant information in an environment which lacks an effective data management policy and a compliance program to hold programs accountable.
The grant programs develop their solution to capture customer data separately in their own silo, and these two different solutions are unlikely to develop the same data standards, data naming conventions, or other aspects of data management that would allow the two programs to integrate easily.
As a consequence, analyzing grant data from the programs for insights on important factors that could make both programs more effective (such as identifying risk indicators that a grantee will fail to deliver) can become significantly more difficult and time consuming.
In this situation, most agencies wanting to adopt data-driven decision-making will turn to creating an analytical platform where data from both programs can be transformed, cleansed, standardized, and integrated. However, this means the development of another data solution with a cadre of data scientists required to curate and normalize the data, which in turn is likely to require a new budget item and new hiring.
Siloed technologies
A second source of pain in an organization with ungoverned data solutions is that the costs of maintaining and supporting these siloed technologies can increase exponentially over time.
Tighter budgets can hinder improvements, debugging, and sometimes basic maintenance, resulting in increasingly poor customer delivery. At this point, the agency may also have realized that while the subject matter of the programs is different, there are significant commonalities among the data services provided that could be streamlined—for example, multiple programs capturing customer data, managing cases, processing benefits applications, and accepting or delivering payments.
Under pressure to reduce costs and improve service, the agency begins looking for ways to centralize its data solutions, only to discover that doing so is expensive, complex, and few of their peer agencies have completed such projects on time and on budget.
Integrating data solutions is significantly more difficult when solutions develop in the absence of an overarching data governance policy and compliance program to help ensure that, as solutions emerge, they are growing in a manner that will be sustainable.
Establishing an enterprise data governance policy is a good first step to bring consistency, interoperability, and other benefits to a data ecosystem, but programs will need to effectively implement that policy in order to create a healthy and sustainable data environment.
Furthermore, if the governance program stops with policy and guidance that merely establishes high-level principles such as “new and existing data systems must adopt standards to ensure data interoperability,” then it is not likely that an actual change will occur in organizational processes or staff behavior.
Sticking with the grant example, for a data governance program to be more effective, it would need to take an active role in designing standards that could work for both grant programs, facilitating discussions and brokering an agreement on the standards for specific required data elements.
It would also need a compliance program with a deadline by which the standards must be adopted to help ensure that those standards were actually being implemented.
Effective compliance
Successful data governance policy programs integrate policy into implementation by using their compliance program as the bridge between policy principles and driving change in data management practice.
Compliance programs achieve this in part by painting a clear picture of what implementation looks like, and does this by establishing concrete expectations of what will be considered successful compliance with policy.
For example, if a data governance policy included a principle of strengthening data quality, the compliance document could articulate that lines of business must establish specific parameters for monitoring data quality aligned with the needs of the program they serve.
A data quality parameter that the compliance program establishes could be that grant recipient payee data will have less than 1 percent of missing values to help ensure timely and accurate delivery of payments.
An effective compliance program should be able to envision a path to evaluate successful compliance. If it cannot do that or needs to rely on a program official’s attestation of compliance, then it is not reasonable to expect the lines of business could comply with a policy directive on their own.
These expectations should be published as part of a compliance policy or procedure so that the lines of business have a yardstick by which to measure their efforts. Ideally, this should focus on the changes the lines of business would need to implement.
For example, a policy principle related to data quality as data is moved across an enterprise might state that data must be complete and maintain its integrity as it moves from one system to another or ingested from an outside source. The compliance program could set criteria that data must be monitored for completeness and integrity such as not incurring any unintended transformations.
The compliance program would further identify any technology performing data movement (including automated data quality monitoring) that would meet this compliance standard, as well as alternative processes a program could implement that would constitute appropriate monitoring of data movement.
This type of information not only paints a clear picture for the program of how they can comply, but also gives them enough information to assess how much effort the program will need to meet the compliance criteria.
‘Easy path’
It is worth noting that the technology and processes cited by a compliance program do not mean that other solutions a program may develop to demonstrate compliance are not to be considered. Rather, the cited technology and process can be examples of an “easy path” to compliance that programs can either adopt or know they already have in place.
In situations where a program develops an alternative approach, the compliance program could evaluate that approach against its criteria—in this case being able to demonstrate that data is moving completely and with integrity.
If the evaluation finds that the program has developed an effective approach, this approach can be added to the compliance program’s list of accepted technologies or processes and shared with other programs as a best practice or, if you will, an additional “easy path” to compliance.
When data governance policy is completed with the publication of a policy document, they can leave program and business leads without direction on prioritization, timelines, and the necessary level of effort and resources needed for implementation.
In many cases, the absence of this information can cause what was previously a willing coalition of business support for better data governance to get cold feet and hinder the passage of new data policies.
A compliance program can help program and business leads set priorities, determine timelines, and plan resources to ensure compliance by adopting and publishing clear criteria for what compliance looks like, as well as a risk-based schedule for its compliance reviews and setting.
Reducing uncertainty for programs regarding how they will be evaluated and what will constitute successful compliance will not only keep a coalition supporting data governance together, but will also drive implementation and change.
Joah Iannotta is Senior Data Governance Expert at ABSG Consulting Inc. Part 2 of this series will explore the practices that data governance compliance programs can adopt to help drive change management.